
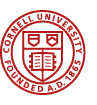
|
GIS Graduate Student Research: Multi-temporal Forest Mapping
Mapping forest communities in the Hudson River Valley using multi-temporal TM imagery
Over the past two decades, many studies have attempted to develop large-area digital maps of vegetation and land cover with varying degrees of success. However, many remote sensing studies in the northeastern United States using single-date imagery failed to accurately depict forested land cover, especially of deciduous types. Recent studies suggest that using a multi-temporal approach to the classification of multi-spectral data may provide greater forest classification accuracy than using a single-date classification. For this study, four reflective bands from four Landsat 5/7 digital images for one TM scene (Path 14 Row 31) were used to create composite images spanning the primary annual variations in the phenology of deciduous forest types in the Hudson River Valley. Classification using a single-date, a 12-band composite (three dates) and a 16-band composite (four dates) image were compared to determine whether forest types can be mapped at a higher thematic accuracy using multi-temporal imagery than using single-date imagery.
Two hundred and forty training sites were identified using a combination of field sampling with GPS and image interpretation of large-scale aerial photographs. Training data were created by expanding training sites into regions, based on the spectral similarity of contiguous pixels. Prior to image classification, randomly selected pixels were removed from training regions and set aside as validation data for accuracy assessment. Using a supervised classification technique, a total of eighteen forest types were classified with Anderson Level III precision.
Overall thematic accuracies for each land cover map were 78.4% (single-date), 89.3% (three-date composite), and 91.7% (four-date composite). Accuracy for the three-date composite (Kappa = 81.2%) was found to be significantly higher than for the single date image (Kappa = 70.0%). Individual accuracies for certain types, especially deciduous forest types, also increased significantly with the addition of multi-temporal data. However, lack of a statistically significant increase in overall accuracy between a three date (Kappa = 81.2%) and four-date (Kappa = 84.6%) classification could imply that there are limits to the benefit of multi-temporal data.
For more information e-mail jenniebraden@hotmail.com.
Resulting publications:
Braden, J. 2002. Mapping forest communities in the Hudson River Valley
of New York State using multi-temporal Landsat TM imagery. M.S. Thesis,
Cornell University, Ithaca, NY.
-

Department of Crop & Soil Sciences
Cornell University is an equal opportunity, affirmative action educator and employer.
|
Research
Outreach
ArcGIS Desktop v.10 Course Descriptions
ArcGIS Desktop I
ArcGIS Desktop II
ArcGIS Desktop III
ArcGIS Web Sessions
Video List
ArcGIS 10 Basics - 1 of 4
ArcGIS 10 Basics - 2 of 4
ArcGIS 10 Basics - 3a of 4
ArcGIS 10 Basics - 3b of 4
ArcGIS 10 Basics - 4a of 4
ArcGIS 10 Basics - 4b of 4
ArcGIS Data Formats - 1 of 2
ArcGIS Data Formats - 2 of 2
Calculating XY Values for a Feature
Clip & Intersect Tools
Connecting to Web Services
Creating a Roster Catalog
Feature Templates 1
Garmin 76SX_Inro
Geodatabase Domains
Geoprocessing Results Window
Layers
Mapping XY Data
|